Gone are the days when scientific progress was confined to individual disciplines, like swimmers rigidly adhering to their designated lanes in a pool. Today, breakthroughs increasingly occur at the intersection of fields, as researchers break free from these traditional boundaries and collaborate across disciplines to tackle complex challenges—from developing personalized cancer treatments based on genomic profiles to creating radiation-resistant materials for space electronics.
Fueling this trend is the rise of high-quality data as the new currency of scientific discovery. The “Big Data” movement has helped boost research by providing scientists with unprecedented access to large datasets. This explosion of data, coupled with advances in distributed computing and frameworks like Apache Hadoop, has enabled data-intensive research and collaboration.
Yet this data-driven research age also presents barriers. “A lot of the problems we’re trying to solve as researchers are complex,” said Jenny Hu, head of customer success and growth at Labstep, a STARLIMS company. “They involve large datasets and many different labs to even get to the point where the data reaches us, the computational scientists analyzing it.”
In addition, this explosion of data and increased reliance on complex analyses has also exacerbated a longstanding problem in science: the replication crisis that persists throughout science. A 2016 paper in Nature noted that seven out of ten researchers have tried and failed to reproduce a colleague’s experiments. And “more than half have failed to reproduce their own experiments,” it noted.
Overcoming data silos can unlock multidisciplinary potential
Despite the popularity of collaboration tools, data often remains siloed within specific disciplines or research groups, hindering cross-disciplinary work. This lack of standardization and interoperability can create significant barriers to integrating data from different sources and gaining a holistic understanding of research questions. For example, biologists studying a particular disease might collect data in a format that is incompatible with the tools and methods used by chemists or physicists working on related aspects of the problem. Biologists, for instance, might store genetic sequencing data in FASTA or FASTQ formats, while chemists might use Crystallographic Information File (CIF) for molecular structures, posing an initial obstacle to collaboration.
For companies, especially those starting early, if they prioritize data as part of their business focus at the C-level, it’s just much easier for this company to then scale.
Jenny Hu, Head of Customer Success & Growth at STARLIMS
In addition, the data-intensive nature of modern research has created a growing demand for scientists with strong coding and data analysis skills. While some researchers are proficient in programming languages like Python, many lack the expertise needed to effectively analyze and interpret datasets so large they are either impossible to open with conventional tools or are simply impractical to analyze without high-performance computing resources.
In a perfect world, researchers would be able easily access and share data across disciplines and organizations in a sort of “shared data universe,” as Barney Walker, Head of Product at Labstep, put it. For example, a pharmaceutical company testing a new drug compound could benefit enormously from knowing if a competitor had already screened that same compound for safety. “And maybe that molecule is just not safe,” Walker pointed out. Such data could prevent years of wasted research and resources.
Yet achieving a truly shared data universe faces numerous obstacles. Concerns about intellectual property protection and data security are significant, as is the sheer volume of data itself. This volume can lead to “data gravity” — the tendency for data to become increasingly difficult to move or share as it grows, hindering collaboration and data integration even within a single organization.
ELNs: Weaving a unified narrative of the scientific process
To address these challenges, tools such as Electronic Lab Notebooks (ELNs) are gaining traction. ELNs offer a centralized, digital platform for capturing, managing, and analyzing research data, supporting collaboration, and enhancing reproducibility. This approach allows researchers to integrate code, data, and narrative in a single, shareable digital environment.
Walker sees lab notebooks as a unifying force in scientific research, connecting diverse data points into a coherent narrative of the experimental process. “What we’re trying to do is be that thread that connects all of those different points of data into a single story,” Walker said. By having a single narrative instead of disparate and disconnected data islands can lead to “a second wave of productivity by integrating tools that were previously separate into one unified workspace,” he said.
This integration and traceability are key features of ELNs. “It’s really obvious that this graph over here came from this code over there, which was using this raw data,” Walker said. “And here are all the steps that went into actually gathering that raw data, and here are all the materials that were used in the experiment. It’s all linked together, so you can just click through everything to see where it came from originally.”
While ELNs support code, users don’t need to be coders to use them. Such notebooks have “run” button functionality for executing code without writing it from scratch. This allows researchers who are not expert coders to still benefit from the power of computational analysis. Moreover, the environment provides access to the underlying code for transparency and customization. Researchers can also leverage a vast ecosystem of libraries, APIs, and tools to integrate specialized analyses, automate workflows, and connect with external data sources, all within the unified ELN environment.
Toward a more open scientific future
Walker envisions a future where this level of transparency and reproducibility becomes the standard for scientific publishing: “Someday in the future, this is how science should be published, right? Not just a block of text in the appendix of a paper, but actually a full, complete digital record of exactly what steps were done and what was used.” This vision aligns with the growing movement towards Open Science, a movement that has grown in traction in the past two decades that advocates for making research data and methods more readily available to the scientific community.
The rise of data champions
As ELNs and other data-driven tools become more prevalent, a shift is occurring in how research teams operate and collaborate. Demand for cloud-based ELN technology, in particular, is strong. These trends are leading to the democratization and broader data sharing. Another driver of data-driven science is the rise of “data champions” within research teams, as observed by Hu. “I notice an increasing trend of data champions within the scientific teams we work with,” she notes. “We call them champion or MVP users — essentially the key individuals that drive adoption within their organization.”
There’s definitely a sense that multidisciplinary research is the future.
Barney Walker, Head of Product, Labstep
While the current wave of interest in AI has democratized coding to a certain extent, that doesn’t mean that scientists are obliged to, say, run Python scripts if they don’t have the time or inclination, Walker said. “You only have to open the full notebook if you’re one of those data champions or more tech-savvy users who are really keen to get their hands dirty with coding,” he said.
Yet the ripple effects of this data-centric mindset are already reshaping the scientific landscape. “There’s also growing awareness of the impact of having good or bad data and understanding how the data is used downstream,” Hu said. “This helps bench scientists appreciate the value of how they capture the data, which then aligns with the processes or data requirements that come later for operating ML models, training algorithms, and facilitating further development in terms of using and leveraging AI.”
This article was written by Brian Buntz, Editor-In-Chief of R&D World.
Or, watch the webinar to learn more…
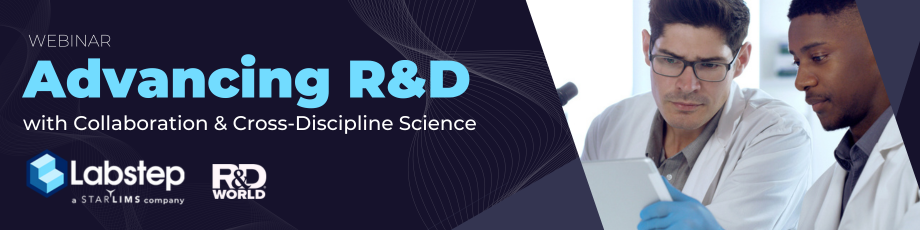